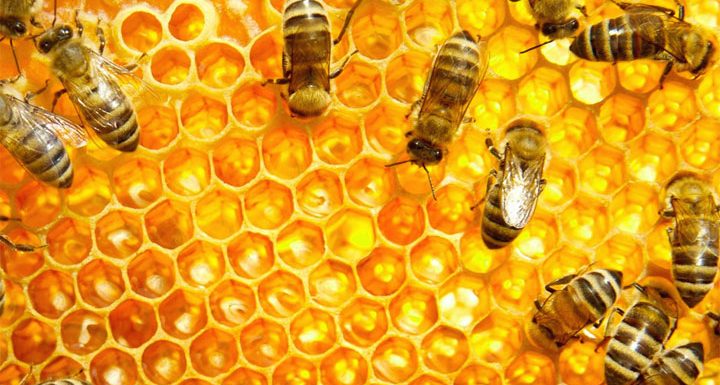
If you try to search for “PSO” abbreviation in wiki you may find Pacific Symphony Orchestra or Phase-shift oscillator. However, my project at HZB is about neither music nor electricity, but mathematics. For almost a month here I have been working on an algorithm called Particle Swarm Optimization method and I would like you to have some impression of it.
Optimization
Actually, the definition of optimization problem is quite simple. One has a function of n-variables f(x1, …, xn) and seeks to find such values of variables that give minimum to the function. However, the solution is not that simple, because in general you cannot use calculus, since the function might be not smooth. That is why we have to look for another approach and here we come to so called Swarm Intelligence (SI) that was originally inspired by social behavior of animals.
PSO method
Imagine how a swarm of bees, for example, would like to solve our optimization problem. Let us assume it is flying in 3-D space and the function represents the density of lilacs in the field. The one who is familiar with biology would say that every honeybee flies around the field remembering its own best position and communicating or sharing it with its sisters through odor cues and dancing. Finally, the optimal location is defined through all these collective work, and everyone flies there. More or less the same technique is applied in Particle swarm method. The basic algorithm works by having a population (swarm) of candidate solutions or particles. Each of them moves around the search space guided by two factors. The first is its personal best position during the previous search. The second is the best known position throughout the swarm. Thereby at some moment there appears a general tendency towards the optimum and the swarm converges on solution.
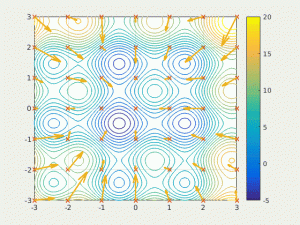

Why BESSY?
You might wonder what all this has to do with accelerator physics and BESSY II. Actually, a synchrotron also needs some optimization and my work here is not only limited with theoretical research of Swarm Intelligence. During the internship I am also implementing the algorithm on python for our colleagues working at BESSY II. Besides, this SI techniques can be extended to multi-objective cases, which means that we can optimize several objectives at once. In particular, it might be applied in accelerators like BESSY II to increase both current and lifetime of the beam.
In conclusion, I would like to add that SI methods may also have applications in other fields like crowd control and robotics. Meantime, it is not a big leap of imagination to picture artificial bees searching for pollen in a field to supply you with pure honey. And you can be sure that they will not bite you.
Thanks! Sure, Rustem, I will tell you everything in details, you can count on me)
It’s beneficial experience for you, my congrats! By the way, I’m just curious if “BESSY” organization has special computing powers? Are you allowed to use it?
I would like to learn more about applications in physics. Notably, how does PSO help to increase current and lifetime of the beam?
That’s interesting. I hope you will continue studying optimisation. If you are interested, you might read about heavy-ball and Nesterov methods. They are quite useful.
It sounds very interesting. I hope you will continue your in the future. And I wait your explanation with more details later;)
Thanks! Will tell you more about it, when I am back)
thanks, Stas!)
Thank you for your question, Aygul! BESSY II is an electron storage ring of 240 meters in circumference with 32 magnets along the ring. These magnets are designed to keep most electrons in a particular orbit, but this aim might be achieved only with nonlinear magnets. However, having these nonlinear magnets it becomes difficult to calculate their impact on electrons (this means that we cannot find an analytical solution of differential equations). That is why we try to find good settings of magnets using optimization methods, i. e. considering the settings as variables and current or lifetime of the beam as an optimised function.
what an awesome post, liked it a lot!
Ilyas, thank you for your interesting and inspirational blog post! In your blog you write about ”BESSY” and i’d like to know more about it!
I am working on it, Fanis! Formally I will present the results in form of poster or talk, but I am also planning to write a blogpost here to cover the main results.
Shall we have a chance to learn your research results?
Nice searching! It is very interesting!
Thank you for your comment, Eli! Actually today there is a whole field of mathematics called Genetic Algorithms which deals with that kind of analogies. The scientists who work on that sort of algorithms try to develop them based on nature inspired methods like mutation, crossover and selection. This is more general but also interesting topic, and I hope may be covered on one of my next posts.
I really like the way you showed the readers how nature and math are linked in such a gracious way. Nice Job!
Thank you for a nice workshop, Antonia! I am really grateful for your quick feedback and help)
Thank you, Dima! That is a tough question, because it really depends on version of the algorithm, but for the basic one we can say that it is O(swarmsize*n + swarmsize*F_cost) for every iteration, where n – is dimension of the problem, F_cost – is computation cost of function. Seems quite fast, but one should take into account that in applications F_cost may play a key role and we have to reduce the number of function computations by any means.
What may be really interesting for you in Swarm Intelligence is the way how particles interact with each other, forming so called different topologies. For example, a particle may receive an information from all particles, but may compare its global best only with its closest neighbours. Changing the topologies you significantly affect the behavior of the swarm and the convergence rate.
Very interesting and well explained!
Thank you, Ilyas! I find your explanation very neat and informative. I wonder how quickly does PS method converge and how hard are the compuations. I meant here what can you say about computational complexity of PS.
Thank you for your question, Alaa. On this contour graph yellow crosses represent the particles (or “bees”) and the vectors – their current velocities. A color bar on the right helps to determine a value of each contour line.
It is only the beginning of the algorithm and particles are quite evenly distributed in space, but you can see a general tendency towards the global minimum in the center.
thanks for that simple explanation, I always find “animal” algorithms interesting to study. Can you explain that contour graph on the right?